Mentoring and Coaching Methodology using Human-Centred Innovation
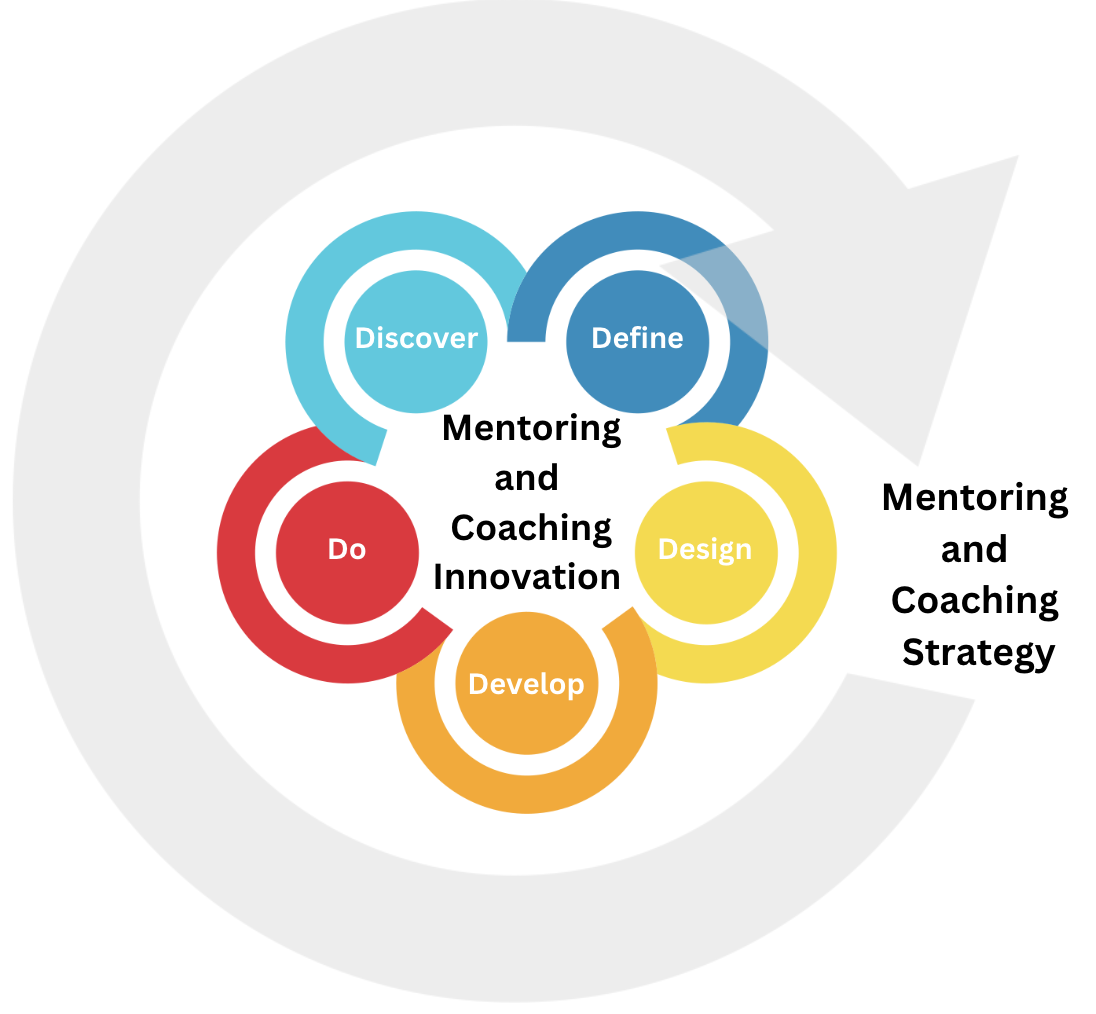
The 5D Framework's human-centred methodology and methods can be used to drive innovative approaches to researcher mentoring and coaching. The actionable insights in the case studies below can be applied to any mentoring and/or coaching scenario.
Case Study 1 Emerging and Early Career Researchers: Mentoring and coaching a research team to find purpose, collaborate, partner with industry and develop a prosperous career platform.
One of the most effective approaches to mentoring is for the mentor to work directly with the mentee on a specific project with clear goals. This enables the mentor to show and tell in situ. These kinds of projects offer real-life scenarios and challenges that research teams can work through together, reflect on, and develop new and better strategies to address. Research challenges/projects provide extraordinary opportunities for developing innovation skills, leadership growth and effective strategies for long-term success.
Case Study 2 Sustainability: Mentoring and coaching a research team to regenerate biodiversity
Case Study 3 Human-Robot Interaction: Mentoring and coaching a research team to Win RoboCup Social Robotics
Case Study 4 Healthcare: Mentoring and coaching a research team to build a trustworthy healthcare device that leverages better AI prediction to make better human-centred decisions
Case Study 1 Emerging and Early Career Researchers
Challenge: Accelerating emerging and early career researchers' development and leadership skills.
A cohort of early career researchers (ECRs) at a university's Artificial Intelligence (AI) research lab is recognised for their high potential. These researchers, from diverse fields such as AI, computer science, cognitive psychology, software engineering, business and law, are engaged in AI research projects. The 5D framework was used to develop a structured mentoring and coaching approach to accelerate their development and innovation skills and cultivate their leadership capabilities.
Discovery: Assessing Individual Strengths and Interests
The mentoring programme begins with a Discovery phase, where the mentor conducts one-to-one sessions with each researcher to understand their strengths, research interests, and career aspirations. The goal is to create tailored development paths and identify opportunities for collaboration within the group.
Focus on Researcher Development:
- Creativity: The mentor encourages researchers to explore topics outside their primary focus areas, such as the intersection of AI and ethics or AI for social good, to broaden their perspectives and stimulate creative thinking.
- Critical Thinking: The mentor facilitates a self-assessment process where researchers evaluate their skill sets and reflect on areas for improvement and development.
Outcome: Researchers set individual development goals, such as publishing in high-impact journals, leading collaborative projects, or presenting their work at global AI conferences.
Define: Creating Personal Development Plans and Setting Milestones
In the Define phase, the mentor works with each researcher to create a personal development plan (PDP) that includes specific, measurable milestones. These goals range from securing research funding to developing novel AI algorithms and contributing to open-source AI projects. The mentor ensures these plans align with both the individual's career goals and the lab's strategic priorities.
Focus on Researcher Development:
- Communication: Researchers present their PDPs to the group, receiving feedback and refining their plans. This process helps them articulate their goals clearly and integrate input from peers and the mentor.
- Leadership: The mentor emphasises self-directed growth by encouraging researchers to take ownership of their development plans and proactively seek resources and support.
Outcome: Each researcher has a detailed PDP, with clear milestones and timelines, enhancing their skills in goal-setting, planning, and communication.
Design: Developing Collaborative AI Research Projects
In the Design phase, the mentor organises interdisciplinary workshops where researchers propose and design collaborative AI projects that leverage diverse expertise within the team. Researchers are grouped based on complementary skills to tackle complex problems such as developing AI models for personalised healthcare or improving NLP algorithms for underserved languages.
Focus on Researcher Development:
- Collaboration: The mentor fosters a collaborative culture, encouraging researchers to work across disciplines and combine their expertise. They learn how to integrate cognitive psychology insights into AI models or apply ethical principles to AI governance projects.
- Innovation: Researchers are encouraged to use innovative methodologies like design thinking, rapid prototyping, and agile development in their projects. They test and refine AI models, exploring novel data sets and techniques to enhance their work.
Outcome: Researchers develop collaborative projects, forming the foundation for publications and grant applications. They gain hands-on experience in managing multidisciplinary projects and leveraging diverse expertise for innovative AI solutions.
Develop: Building Technical and Leadership Competencies
In the Develop phase, the mentor emphasises building technical competencies and leadership skills. Researchers work intensively on collaborative projects, applying their PDPs and taking on leadership roles. The mentor provides targeted technical support, helping them refine methodologies, improve data analysis techniques, and optimise AI algorithms. Leadership coaching focuses on project management skills, effective team communication, and constructive feedback.
Focus on Researcher Development:
- Leadership: Researchers participate in leadership workshops where they practise managing project timelines, coordinating tasks, and making strategic decisions. The mentor rotates leadership roles to provide experience in different team dynamics and responsibilities.
- Teamwork: The mentor facilitates group reflection sessions, where researchers discuss challenges and achievements. These sessions foster a culture of transparency, accountability, and mutual support within the team.
Outcome: Researchers gain confidence in managing and leading AI projects, developing stronger technical and leadership skills that enhance their ability to deliver impactful AI solutions.
Do: Showcasing and Expanding Research Impact
In the final Do phase, the mentor helps researchers showcase their work and expand their professional impact. The focus is on preparing for conferences, writing grant proposals, and networking with industry experts and senior academics. The mentor supports researchers in developing strategic career plans, guiding them on the next steps to secure research funding, publish influential papers, and build international collaborations.
Focus on Researcher Development:
- Communication: Researchers receive coaching on presenting their work effectively to diverse audiences, including technical and non-technical stakeholders. They practise public speaking in mock settings, refining their ability to explain complex AI concepts in an engaging and accessible way.
- Professional Growth: The mentor introduces researchers to key industry partners and academic networks, encouraging them to present at conferences and engage with the AI community to enhance their visibility and professional connections.
Outcome: Researchers successfully present at major AI conferences, secure grants, establish new collaborations, receive invitations to engage with industry and are in demand to lead research and innovation in industry and at universities. They gain recognition in their fields, accelerating their career trajectories and contributing to the lab's reputation as a leader in AI research.
Summary
This case study demonstrates how a structured mentoring and coaching approach can accelerate the development of emerging researchers in the AI field. By focusing on creativity, critical thinking, communication, collaboration, and leadership development, the mentor empowered the researchers to become confident leaders and innovators. The combination of personalised development plans, interdisciplinary collaboration, and practical leadership training enabled the team to achieve significant research outcomes while building the skills necessary for long-term success in AI research.
Case Study 2
Sustainability: Mentoring and coaching a team to regenerate biodiversity
Challenge: A high-performance research team focused on trustworthy AI for environmental sustainability in collaboration with the Borneo Nature Foundation, local forest, flora and fauna rangers, and the Indonesian government. The mentor used the 5D Framework to enhance the creativity, innovation, critical thinking, communication, collaboration, and teamwork of the ECR, Post-Doctoral, Postgraduate and Undergraduate researchers. The goal of this project was not only to deliver a cutting-edge, trustworthy AI but also to develop the researchers' skills and mindsets as collaborative innovators and leaders.
Discovery – Fostering Creativity and Critical Thinking
In the Discovery phase, the mentor emphasises the importance of divergent thinking. Rather than jumping straight to solutions, the researchers are encouraged to explore wide-ranging perspectives on environmental sustainability. They engage in brainstorming sessions where each team member presents research insights and potential innovative directions.
Focus on Researcher Development:
- Innovation: The mentor organises activities like "What If" scenarios where researchers imagine radical approaches to park management using AI.
- Critical Thinking: The team conducts peer reviews of each other's research findings to challenge assumptions and ensure rigour.
Outcome: Researchers expand their thinking beyond conventional AI applications, exploring possibilities such as AI-driven biodiversity monitoring and dynamic predictive models for ecosystem management.
Define – Enhancing Communication and Critical Thinking
The Define phase focuses on synthesising the creative ideas generated in Discovery into a clear research direction. The mentor guides the team in narrowing their focus through structured debates and discussions. They practice framing the problem and potential solutions in a way that is compelling to diverse stakeholders (e.g., policymakers and ecologists).
Focus on Researcher Development:
- Communication: Each researcher must present a defined research question and defend it during a team debate. They must also explain their ideas to non-expert stakeholders, promoting clarity in their communication.
- Critical Thinking: The mentor uses the "Five Whys" technique to ensure the team critically evaluates the root causes of the problem they aim to solve.
Outcome: The team collectively agrees on a key focus—an AI system that predicts illegal activities and monitors biodiversity impacts.
Design – Encouraging Collaboration and Innovation
In the Design phase, the mentor introduces methods like co-creation workshops, where the team works together to brainstorm and design potential solutions. To ensure collaboration, researchers are divided into cross-disciplinary pairs, forcing them to work outside their comfort zones and integrate diverse perspectives.
Focus on Researcher Development:
- Collaboration: The mentor facilitates a design sprint where each team member leads a part of the session. The rotating leadership structure helps them develop collaborative leadership skills and the ability to work with different personalities.
- Innovation: Researchers are tasked with designing novel prototypes or frameworks based on a combination of AI and ecological science. They iterate through sketches and low-fidelity models.
Outcome: The researchers develop a prototype AI tool that leverages real-time environmental data to provide adaptive park management strategies, driving innovation within the field.
Develop – Building Teamwork and Communication
As the researchers move into the Develop phase, the mentor focuses on effective teamwork and agile communication practices. The team works in small, self-organised groups, but regular check-ins ensure that communication remains fluid and transparent across the team.
Focus on Researcher Development:
- Teamwork: The mentor introduces collaborative tools like shared dashboards and collaborative coding environments. Researchers are encouraged to provide real-time feedback on each other's progress.
- Communication: Weekly "demo days" allow each sub-group to present their progress, helping researchers sharpen their ability to present technical ideas clearly and receive constructive feedback from peers.
Outcome: The team refines their AI tool, with each iteration benefiting from improved communication and collaboration across disciplines, driving a collective sense of ownership over the project.
Do – Leadership, Collaboration, and Professional Growth
In the Do phase, the research team implements the AI tool across select national parks. This phase challenges the researchers to engage with external stakeholders, ensuring their solution meets real-world needs. The mentor shifts focus to developing leadership qualities and fostering collaboration with external teams (e.g., government agencies and NGOs).
Focus on Researcher Development:
- Leadership: Each researcher is assigned a leadership role in an aspect of the project rollout (e.g., stakeholder engagement, technical refinement). They must coordinate efforts and ensure alignment with the overall project goals.
- Professional Growth: The mentor encourages reflective practice, where researchers document what they've learned and how they've grown as innovators throughout the project. They also present their research findings at an international conference, sharpening their public speaking and professional networking skills.
Outcome: The AI tool is successfully deployed, and the researchers have developed a rich set of skills in leadership, collaboration, and communication. Their professional profiles are elevated as a result of their deep engagement in the project.
Summary
The Innovation 5D Framework not only helped the team achieve a tangible research outcome but also transformed their approach to research. By focusing on creativity, critical thinking, collaboration, communication, and teamwork, the mentor developed each researcher's capacity to innovate, lead, and collaborate effectively across disciplines. Through iterative feedback, hands-on workshops, and structured communication, the team emerged with strengthened interpersonal skills and a greater ability to drive future innovations.
Case Study 3
Social Robotics Mentoring and Coaching a Team to Win RoboCup, the leading International Robotics Competition
A diverse research team at a university is preparing to compete in the prestigious RoboCup Social Robotics competition. Their challenge is to design and program a social robot that can interact naturally with humans in real-world scenarios, such as assisting in a public environment or performing tasks in healthcare settings. The team comprises AI experts, roboticists, human-computer interaction (HCI) specialists, and behavioural scientists. The mentor's role is to coach the team through the technical, strategic, and collaborative aspects of the competition using a structured approach.
Discovery – Cultivating Creativity and Critical Thinking
In the Discovery phase, the team is encouraged to explore the key challenges in social robotics. They conduct user research to identify where social robots could provide the most value in daily interactions, focusing on tasks like assisting elderly individuals or answering questions in public spaces. The mentor emphasizes the broad exploration of user needs and challenges the team to think creatively about the potential of robots in social contexts.
Focus on Researcher Development:
- Creativity: The team is guided through brainstorming exercises to generate novel ideas for how the robot could respond to human emotions, handle social cues, and manage complex interactions like conflict resolution.
- Critical Thinking: The mentor introduces methods like root cause analysis to help the team critically evaluate which robot behaviours and interaction models would provide the best user experience, balancing technical feasibility with user desirability.
Outcome: The team identifies an opportunity to focus on developing a social robot that can assist individuals with cognitive impairments in daily tasks, using natural language processing (NLP) and emotional recognition.
Define – Enhancing Communication and Problem Solving
In the Define phase, the team works to clearly outline the problem they aim to solve. The mentor emphasizes the importance of effective communication to align the multidisciplinary team and clarify their shared goals. They define the key capabilities their robot will need, including real-time emotion detection, context awareness, and voice interaction.
Focus on Researcher Development:
- Communication: The team participates in structured workshops where they must communicate their ideas clearly across disciplines. The mentor encourages concise presentations and the use of storyboards to illustrate complex robot behaviours and interactions.
- Critical Thinking: They engage in detailed problem-framing sessions, where each feature of the robot is broken down to assess its necessity and impact. The mentor also introduces hypothesis testing to validate their design decisions before moving into the prototyping stage.
Outcome: The team finalises a clear problem statement: "How can we develop a social robot that provides empathetic support to users with cognitive impairments, improving their ability to perform daily tasks independently?"
Design – Fostering Collaboration and Innovation
In the Design phase, the team starts developing the robot's core features, such as its NLP model, emotional recognition system, and physical behaviours. The mentor introduces collaborative design processes to ensure cross-disciplinary integration. They hold co-creation sessions where roboticists, AI specialists, and HCI experts come together to design the robot's interface, responses, and physical interactions.
Focus on Researcher Development: Focus on Researcher Development:
- Collaboration: The mentor encourages team members to work in cross-functional subgroups, where each group is responsible for designing a different aspect of the robot (e.g., voice interaction, facial recognition, movement patterns). Regular feedback sessions are held to ensure everyone's work aligns with the overall project vision.
- Innovation: The team is tasked with creating low-fidelity prototypes, testing different AI algorithms, and simulating human-robot interactions. They experiment with voice recognition technologies and adaptive machine learning models to make the robot more responsive to emotional cues.
Outcome: The team designs a robot prototype capable of engaging in basic conversations, recognizing a limited set of emotions, and assisting users by offering personalised reminders and emotional support.
Develop – Building Teamwork and Iteration
In the Develop phase, the mentor focuses on helping the team refine their social robot through iterative testing. Agile methodologies are introduced, with the team working in short sprints to continuously improve the robot's interaction capabilities and overall functionality.
Focus on Researcher Development:
- Teamwork: The team adopts agile workflows, with daily stand-ups and sprint reviews to ensure they are aligned and making progress. The mentor fosters a sense of shared responsibility, encouraging team members to take ownership of different aspects of the robot's development.
- Communication: The team presents their progress in regular "demo days," where they receive feedback from external judges and stakeholders. The mentor coaches the researchers on how to effectively present technical details while communicating the robot's real-world impact in an engaging manner.
Outcome: The robot's performance improves significantly, with better voice recognition accuracy and more natural emotional responses. The team also refines the robot's ability to learn from user interactions, enhancing its adaptability.
Do – Leadership, Collaboration, and Performance under Pressure
In the final Do stage, the team prepares for the RoboCup competition. The mentor shifts focus to honing leadership skills and ensuring the team is mentally and strategically prepared for the high-pressure environment of the competition. Team members are encouraged to take on leadership roles, managing different aspects of the robot's performance and deployment.
Focus on Researcher Development
- Leadership: The mentor assigns leadership roles within the team, allowing each member to lead a key aspect of the robot's performance (e.g., speech generation, user interaction scenarios). This fosters a sense of accountability and leadership growth.
- Collaboration: The mentor emphasises the importance of collaboration during the competition, ensuring that the team communicates effectively and remains agile in responding to real-time challenges during the competition.
- Professional Growth: The team engages in reflective sessions to assess their learning journey and document how their teamwork, creativity, and problem-solving skills have grown throughout the project.
Outcome: The team wins the RoboCup Social Robotics competition, with their social robot excelling in human-like interaction, emotional recognition, and task assistance. The team members develop stronger leadership skills, collaborative abilities, and technical expertise in AI and robotics. Their success is also attributed to their strong communication, teamwork, and innovative approach to solving real-world problems.
Summary
The Innovation 5D Framework provided the structure and guidance needed to coach a high-performance research team to victory in the RoboCup Social Robotics competition. By focusing on creativity, critical thinking, communication, collaboration, and leadership development, the mentor helped the team achieve technical excellence and grow as professionals capable of driving future innovations in social robotics.
Case Study 4
Healthcare Mentoring: Build a healthcare device that leverages better prediction to make better decisions
A high-performance research team in a leading healthcare institution is tasked with developing an AI-powered wearable device to monitor and predict heart health. The team consists of data scientists, medical professionals, and engineers. A mentor is brought in to guide the team using the Innovation 5D Framework to ensure the project advances while also focusing on developing the team's creativity, innovation, critical thinking, communication, collaboration, and teamwork.
Discovery – Encouraging Creativity and Critical Thinking
In the Discovery phase, the research team explores the landscape of heart health monitoring, looking at current technologies, patient needs, and areas where AI can add the most value. The mentor encourages the team to think broadly about potential applications for AI in early detection and real-time monitoring of heart conditions.
Focus on Researcher Development:
- Creativity: The mentor introduces creative ideation techniques like mind mapping and scenario planning, pushing the team to explore unconventional applications of AI in healthcare, such as AI-assisted behavioural tracking that links heart health with lifestyle choices.
- Critical Thinking: The team conducts a systematic review of existing heart monitoring devices, identifying gaps and opportunities for innovation. They evaluate the ethical implications of AI in healthcare, prompting deep discussions on data privacy and patient safety.
Outcome: The team identifies a core opportunity: developing an AI-powered wearable that not only monitors real-time heart activity but also predicts potential heart risks based on patient-specific data.
Define – Building Communication and Critical Thinking
As the team transitions to the Define phase, the mentor emphasises the importance of clear communication. The team narrows their focus to developing a predictive AI model for atrial fibrillation (AFib), a common but often undiagnosed heart condition.
Focus on Researcher Development:
- Communication: Each team member presents their ideas, refining the problem scope. The mentor introduces techniques like the elevator pitch, where researchers must communicate their concept in under a minute to ensure clarity and conciseness.
- Critical Thinking: The team engages in structured debates, examining the technical feasibility, ethical challenges, and medical accuracy of various approaches to AI-powered heart monitoring. They perform a root-cause analysis to ensure their solution targets the correct problem.
Outcome: The team agrees on a focused research question: "How can AI accurately predict atrial fibrillation based on continuous monitoring data while ensuring patient trust and data security?"
Design – Promoting Collaboration and Innovation
In the Design phase, the team moves from problem definition to solution generation. The mentor organises collaborative design sprints where cross-disciplinary pairs work together to propose different AI algorithms and hardware solutions for the wearable device.
Focus on Researcher Development:
- Collaboration: The team works in cross-functional groups, blending expertise from different disciplines. The mentor encourages open communication through daily standups and design workshops where feedback is continuously shared.
- Innovation: The researchers explore different machine learning models and sensor technologies. They are tasked with designing low-fidelity prototypes and experimenting with unconventional data inputs like movement patterns and sleep cycles to enhance the device's predictive capabilities.
Outcome: The team designs a prototype AI wearable that integrates real-time heart monitoring with predictive analytics. The device features both standard heart metrics and novel inputs, such as sleep data, which enhances its ability to predict AFib occurrences.
Develop – Enhancing Teamwork and Communication
As the team progresses into the Develop phase, they focus on building the prototype and testing its effectiveness. The mentor reinforces the importance of teamwork and effective communication, using agile methodologies to maintain momentum.
Focus on Researcher Development:
- Teamwork: The team adopts agile working methods, breaking the project into small, manageable sprints. Each sprint ends with a review session where every member contributes feedback on the AI model's accuracy and the wearable's usability.
- Communication: The mentor introduces the team to "demo days," where they showcase their progress to external stakeholders, including medical professionals and potential investors. Researchers develop their public speaking skills, learning to present complex technical details in an accessible manner.
Outcome: The prototype is refined through iterative testing, increasing the AI model's prediction accuracy by 18% compared to current heart health wearables. The team improves the device's user interface to ensure patient engagement and ease of use.
Do – Leadership, Collaboration, and Professional Growth
In the final Do stage, the team prepares to pilot their AI-powered heart monitoring device in clinical settings. The mentor shifts focus to leadership development and external collaboration as the team works with hospitals and healthcare professionals to deploy the device.
Focus on Researcher Development:
- Leadership: Researchers are assigned leadership roles in different aspects of the project—clinical testing, patient onboarding, and AI optimisation. They must guide their teams and report back on the pilot's progress.
- Collaboration: The team collaborates with doctors, nurses, and patients to gather real-time feedback and iterate the device further. They engage in reflective practice, discussing how the AI system performed in real-world conditions and where improvements can be made.
- Professional Growth: The mentor encourages each researcher to present their findings at healthcare conferences and write about their work in peer-reviewed journals, solidifying their reputation in both AI and healthcare fields.
Outcome: The AI device is successfully piloted in two major hospitals, with initial results showing improved detection rates of AFib in high-risk patients. The team's work is recognised with a healthcare innovation award, and the researchers advance their careers by gaining invaluable experience in leadership, collaboration, and cutting-edge healthcare innovation.
Summary
The Innovation 5D Framework helped guide the development of an innovative AI-powered heart health device while fostering critical skills in the research team. By focusing on creativity, innovation, critical thinking, communication, collaboration, and teamwork, the mentor ensured that the researchers grew as scientists, innovators and leaders in healthcare AI. The structured but flexible framework allowed the team to navigate complex challenges, produce a novel solution, and achieve real-world impact.